In-Situ Metrology and Machine Learning Modeling for Additive Manufacturing
Recent attention by the aerospace industry has focused on additive manufacturing (AM) of metallic components where the advantages are poised to significantly transform aircraft and spacecraft propulsion and eventually other high-value and/or complex components of these vehicles. A primary challenge in achieving these goals is the flight worthiness certification of AM constructed components. Lack of quality assurance with AM parts is a critical barrier in the aerospace industry where component failure cannot be tolerated. In fact, this is the primary issue limiting the widespread adoption of AM components by any industry. Current qualification and certification approaches are inadequate to capture the wide variety of AM machine types and the vast parameter space created by them. In order for this disruptive technology to transform the manufacture of complex metallic components, significant advancements in in-situ process monitoring and control are required to standardize production and certification methods.
This NIST funded project focuses on developing an integrated tool for in-situ monitoring of metals based AM processes. The main objective of the modeling, monitoring and control aspect of the project is to build various data-driven and hybrid machine learning (ML) models, including deep learning (DL) models, to infer the final product qualities from in situ sensing data, and to ultimately achieve real-time control of final product quality by manipulating operation parameters.
Data fusion of all information gathered will be performed towards developing models that infer the final product qualities from in situ sensing data, and to ultimately achieve real-time control of final product quality by manipulating operation parameters. Enabling smart or advanced manufacturing (aka Industry 4.0 in EU) has drawn increased attention recently, especially in the U.S. (e.g., initiatives such as National Network for Manufacturing Innovation (NNMI)). One of the focuses of smart manufacturing is to create manufacturing intelligence by equipping/instrumenting manufacturing systems with advanced sensing and characterization tools for collecting real-time data to support accurate and timely decision making, such as process monitoring and control. Because additive manufacturing (AM) technology is still in its early stages of the development, it is behind some industries (e.g., semiconductor manufacturing) in terms of in-situ sensing and process monitoring/control. However, because of the great potential of AM, we believe that AM equipped/integrated with advanced sensing and characterization tools has the potential to be at the forefront of smart/advanced manufacturing.
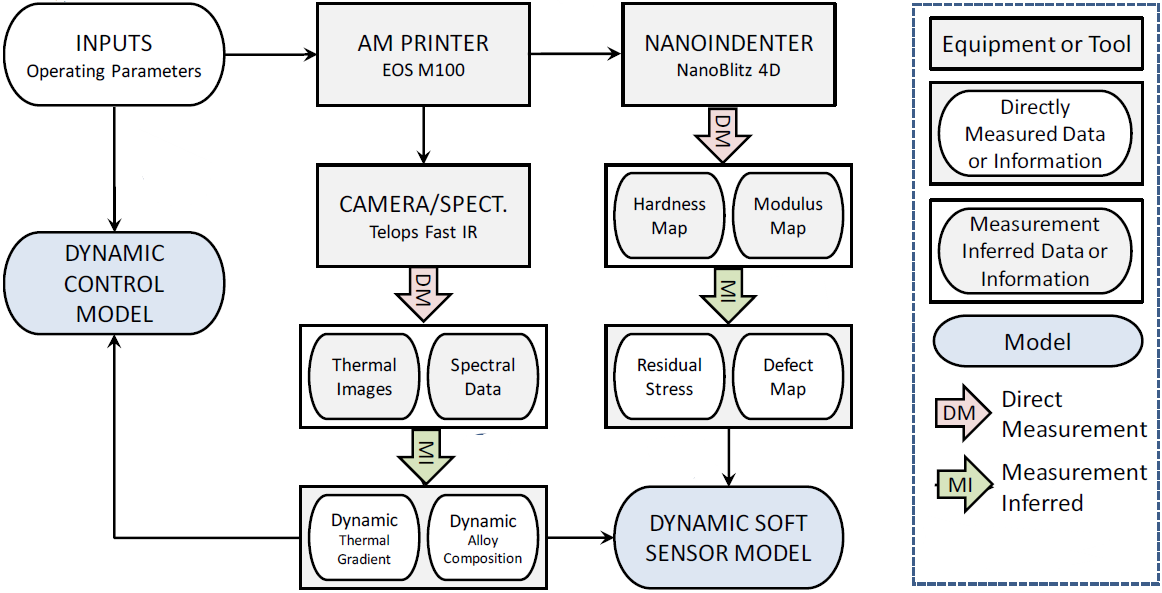
Various ML models will be developed to infer thermal gradient, alloy composition, area of residual stress, location of critical defects etc.
Dynamic soft sensor ML/DL models will be developed to correlate the final product quality (i.e., residual stress, defects, etc.) with the in situ measurements inferred process information (i.e., spatial and temporal thermal gradient mapping, alloy composition, etc.). This is a highly challenging task as the manufacturing steps are highly dynamic and nonlinear. Although the information on the thermal gradient and alloy composition will be obtained at high frequency in real-time, the information on the residual stress and defects is only available after the step (i.e., layer) is completed. Therefore, from the dynamic modeling perspective, dynamic, nonlinear ML/DL models are needed to take the time series input of thermal gradient and alloy composition to predict the product quality at the end of the processing step. Similar modeling challenges exist in the semiconductor manufacturing and chemical processes, and Dr. He has developed various tools to address them. Those tools will be adapted to this project, and new approaches will be developed to address the unique characteristics associated with AM. The key question to be answered in this task is whether the spatial and temporal thermal gradient and alloy composition mappings can provide sufficient information to uniquely and accurately predict the final product quality, and if not, what additional information is needed to help improving the model predictability.