Next-Generation Process Monitoring Techniques for Smart Manufacturing
The goal of process monitoring is to detect the onset and identify the root cause of any change that causes a manufacturing environment to deviate from its desired operation. Process monitoring is an important component and key enabler for the long-term reliable operation of any manufacturing processes. In the era of smart manufacturing, the importance of process monitoring can only become greater when the controlled systems are getting more complex, equipped with more sensors, operated under non-steady state, controlled at tighter margins, and getting yet closer to autonomous operations.
The aim of our research is to create manufacturing intelligence from real-time data for process monitoring. Our approach integrates systems engineering principles such as dynamic modeling and optimization with data analytics techniques such as statistical approaches,artificial neural networks, and deep learning algorithms. In other words, our approach is an integrated human intelligence and artificial intelligence (HI-AI) approach that takes advantages of the latest advancements in these fields.
This National Science Foundation funded project is a synergistic collaboration between Auburn and Praxair(now Linde). Both Auburn Chemical Engineering and Praxair are national leaders of smart manufacturing with different strengths. Praxair is a very energy intensive business –over $1B on energy usage every year in the US alone just to run facilities. Anything can be done to save energy (electricity, natural gas, etc.) is highly desired. If successful, this project will enhance Praxair’s ability to monitor different plants worldwide from a centralized operating center in the US.The outcomes of this project have the potential to help shape the future of smart manufacturing, which is the next wave of innovation in manufacturing worldwide.
This collaborative project will expose Auburn graduate and undergraduate students to current and future industrial needs and provide opportunities for interacting with industrial researchers. These experiences will help prepare valuable future work forces with big data analytics skills that will become critically needed for smart manufacturing in various industries and businesses.
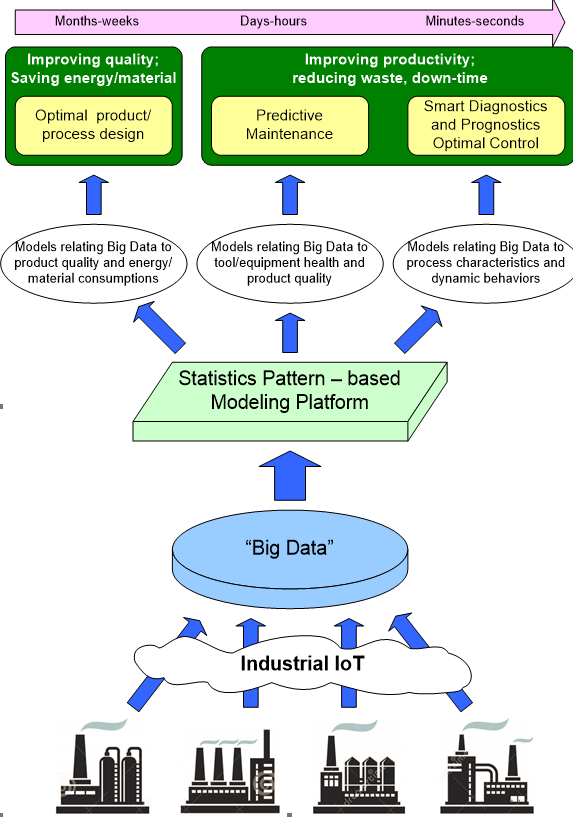