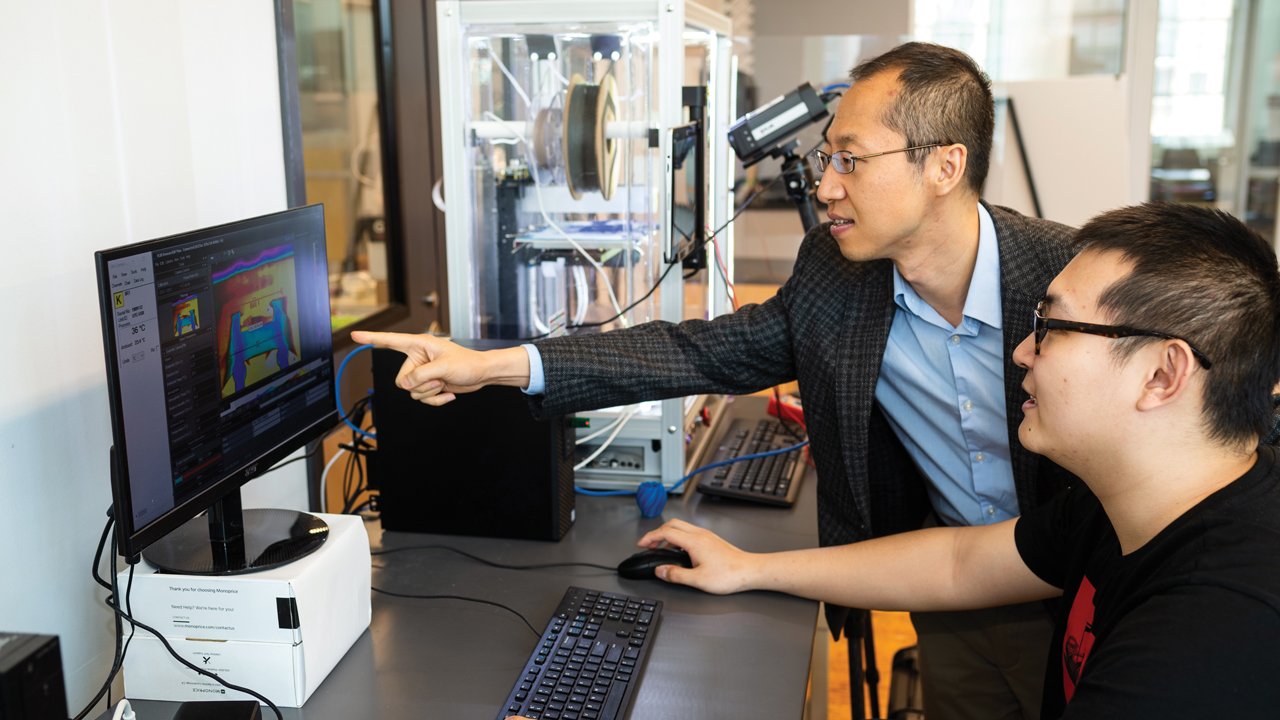
Five years ago, GE Aerospace transitioned to using additive manufacturing (AM) for its new catalyst turboprop engine, which meant that 800 traditionally manufactured components were consolidated into just 12 metal AM parts. In that time, the development cycle was dropped to two years, the engine weight reduced by 5% and the fuel consumption improved by 1%. For G.E., that’s a big deal.
If other U.S. aircraft manufacturers went the same route, that would be big deal for the planet.
There’s a reason AM research across the globe is expected to push $24 billion by 2027, and the revolutionary impact on the aviation industry is one of them. Experts estimate that substantial adoption of 3D-printed parts could knock 200 million gallons off of annual aviation fuel consumption. If the parts work.
Despite the demonstrated success of metal additive manufacturing in various industries, the performance uncertainty of AM parts undermines the potential of deploying AM for high-consequence applications, such as air travel and space travel.
That is why the National Science Foundation is turning to Peter Liu, assistant professor of industrial and systems engineering, in the form of a $500,000 CAREER Award meant to generate new insight into defect formation relevant to fatigue performance of parts manufactured through laser powder-bed fusion and uncover the synergistic impacts of multi-scale factors on fatigue fractures. Liu’s project will establish a physics-centric, machine learning framework for fatigue life predictions, serving as a technological foundation for future metal AM production of dynamic load-bearing applications and establishment of a resilient and reconfigurable supply chain, enhancing the competitiveness of U.S. industry.